Do you focus on brand awareness? Remember that consumers make or break your brand. One of the best marketing strategies for customer engagement and brand awareness is online advertising. No doubt, that Google is the giant advertising platform for small, medium and large firms, but still there are some brilliant opportunities that you might be missing out on. Ever heard of Amazon marketing? Well, you might have heard about Amazon, but are you using Amazon to generate leads? Dig in to know why you should make Amazon part of your advertising strategy.
Most CEOs recognize the power of data-driven transformation. They certainly would like the 20% to 30% EBITDA gains that their peers are racking up by using fresh, granular data in sales, marketing, supply chain, manufacturing, and R&D. And they may even dream of joining the ranks of data-driven companies that have shoved aside traditional players among the world’s most valuable companies. (See Exhibit 1.)
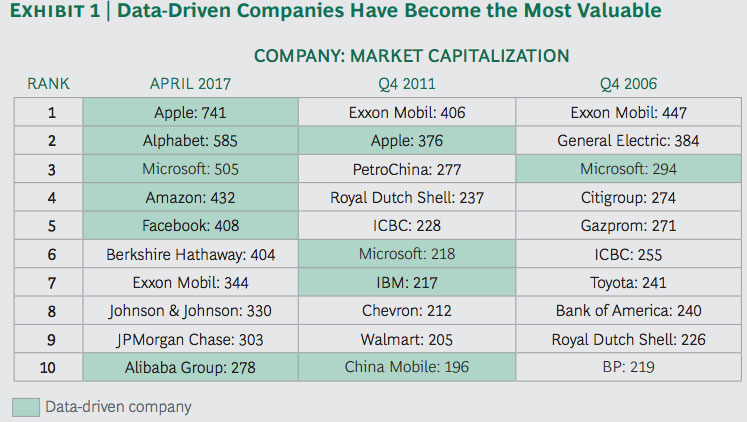
Yet CEOs are right to wonder how their organizations—where managers and executives already complain about a lack of data skills and where overburdened IT systems seem unlikely to be able to handle a tenfold increase in company data—can pull off such a transformation. These CEOs want to find a reliable way to move their companies into the data-driven future so that they can set up their companies to survive—and not put them in danger in the process.
There is a better way to approach data transformation. In our experience, these initiatives can succeed only if they are cost-effective, incremental, and sustainable. Transformations should start with pilots that pay off in weeks or months, followed by a plan for tackling high-priority use cases, and finishing with a program for building long-term capabilities. Working with clients across industries, we have developed a three-phase approach to data-driven business transformation. It starts with small-scale, rapid digitization efforts that lay the foundation for the broader transformation and generate returns to help fund later phases of the effort. (See Exhibit 2.)
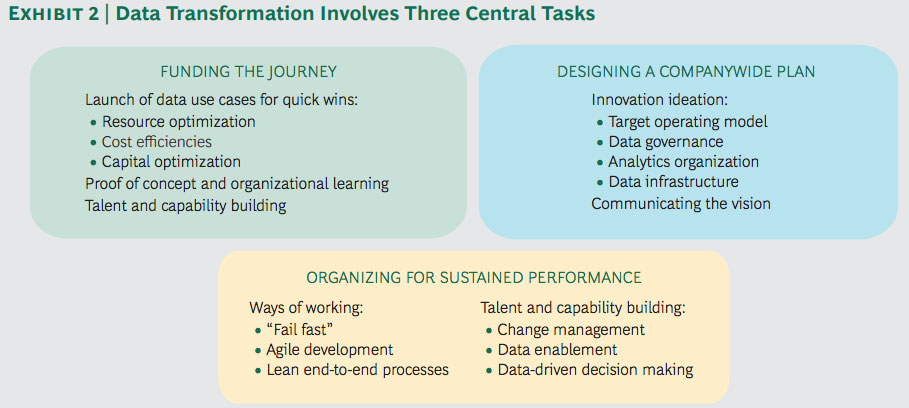
Five critical steps for a successful data transformation
The assessment of data capabilities gives the company the information it needs to carry out five critical steps.
Step 1: Understand where you are on the analytics journey
Organizations slow to derive value and insight from their data risk being at a significant competitive disadvantage. Part of their response must be in advancing their analytics capabilities, an approach that has technical, skills-based, and cultural elements. Data-centric competencies and knowledge need to be built on collective experience, individual skills, and organizational maturity. To address these issues, step one is about evaluating your current analytical state and the value it delivers to users.
From a technology perspective, we can grade analytics capabilities into five levels, starting with passive, descriptive analytics through to machine-driven, cognitive analytics (see Exhibit 3).
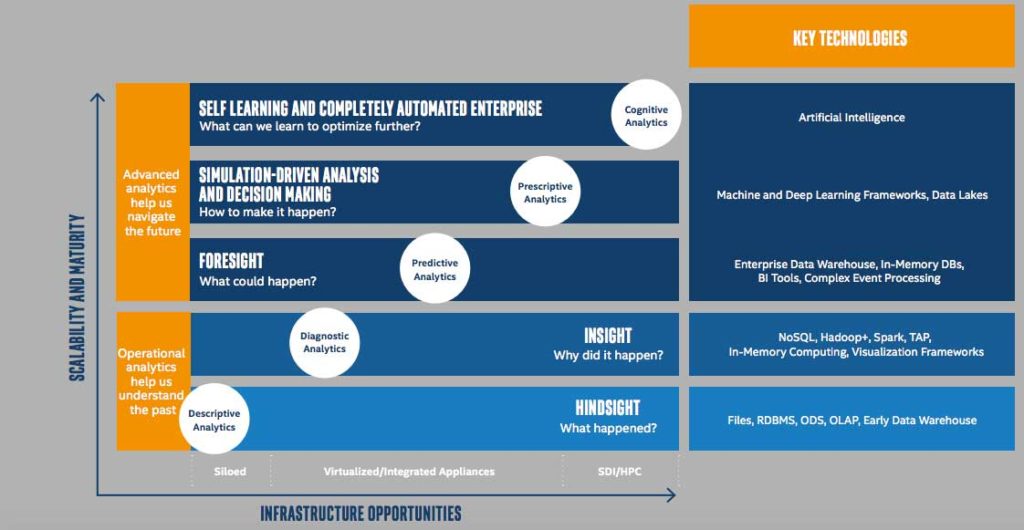
Step 2: Understand business drivers and transformative outcomes
Whatever business models or use cases your analytics initiatives focus on, the task of selecting and prioritizing them must be led by your business peers. It is up to them to decide which opportunities to pursue, with technology experts as facilitators. This stage, while necessary, can be challenging, as it may require changes to business strategy.
Having identified business priorities, you can set out a data strategy focused on the future of the business, rather than its past. As IT, your job is to equip your organization with the most efficient mechanisms and technology platforms to capture, store, analyze, and secure your data so insights can be delivered rapidly and scaled across the breadth of your organization. Business priorities set both a delivery roadmap and scope so, for example, some of the data that you have so carefully been collating and managing may no longer be applicable to the business’s needs. Equally, you will find that you need to gain access to previously untapped sources of data.
At the same time, the business will need to accept approaches that put data front and center of business practice. For example, it may be more appropriate to create a data portal accessed by front-line staff rather than managers: such changes may be empowering to sta, but may also create organizational or political concerns (which could be expressed in questions of governance or security). It is therefore very important to start with a deep and senior level of business buy-in to data-centricity. IT executives can help these leaders understand the opportunities by:
- Running facilitated sessions with senior executives to explore opportunities and set priorities for higher-order analytics
- Providing insight and input, based on what is happening elsewhere in the industry, from some of the more advanced players or disruptive startups
- Demonstrating the financial benefits of adopting data-driven approaches, through an overall business case – but this can only exist as a precursor to the business deciding its strategy
- Promoting success stories in more data-centric lines of business, showing where the use of more analytical practices has been successful for your enterprise
Step 3: Create a data-centric foundation architecture
Once both the goals and the current state of readiness to meet them have been established, IT can plan the infrastructure to support transformation. Modernizing the data center with highly-optimized infrastructure across every critical domain (compute, storage, network, and security) is a foundational step for longer-term analytics success. However, it isn’t necessary to do it all at once. Nor would this be a good idea: today’s untethered data lives everywhere — in your data center, in the cloud, and in connected devices sitting at the edge of your network. From a technological perspective, the priority is to establish the data architecture your organization will need. Step two will have revealed the organization’s strategy for data-centricity, where to prioritize efforts, and the scope of the data. involved. Then you can take a more comprehensive view of the distributed data model you are looking to employ, covering how data arrives into and is shared across your organization, kept secure, and analyzed to drive insights where your business needs them.
Various platforms are available today to help enterprises get beyond “rearview mirror” reporting and traditional BI to more predictive capabilities and real-time insights powered by advanced analytics and machine learning. However, it is not necessarily about one solution over the other, but more about having the right toolbox and,
knowing which tool to use in which scenario. This toolbox will likely contain a combination of technologies and services, public and private, in-house and from partners, modern and legacy, delivered based on a service platform and an API-driven service layer (see Exhibit 4). As you review the tools you will need, you can rank them according to the following criteria:
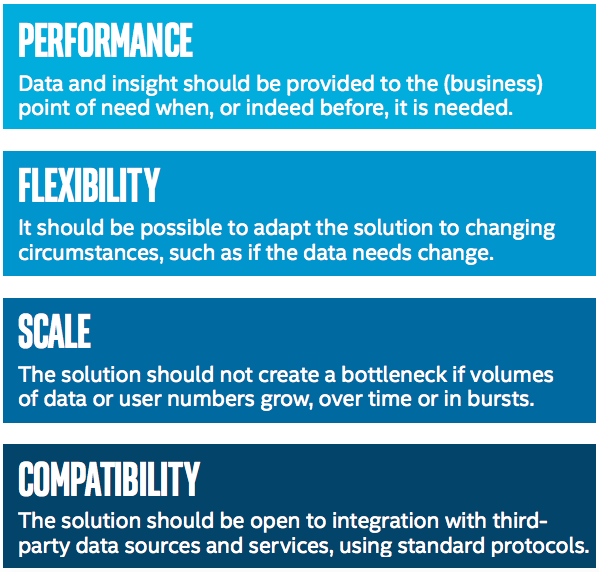
In parallel with defining a forward-looking distributed data architecture, you can consider the processes and relationships required to make the most of a data-driven environment. The additional goal of this step is to make sure the IT organization is aligned with enterprise teams, focusing on analytics and thinking about how to meet business goals. Rather than making data the responsibility of IT or business analysts, the aim should be to show that data is key to empowering better decision-making at every level of the organization.
Once you have a solid picture to build on, you can test it out with a well-supported proof of concept (PoC).
At the bottom line, Amazon marketing is a great way to reach out to a global audience. It is the gold mine to find your prospective consumers. So, wake up! Spread your business worldwide!
Step 4: Create a PoC to prepare for a bimodal future
The primary goal of analytics practitioners today is to support lines of business to deliver competitiveness using insight. However, you don’t have to make big investments in infrastructure immediately. You can adopt data-driven approaches that ensure the benefits outweigh the costs from day one, enabling you to get the basics right while creating capacity so you can start building for the future.
The goal of a PoC is not only to test your models and ideas but also to set the scene for a bimodal future in which you encourage innovation and experiment in parallel with assuring service levels and minimizing risk. Our experience suggests that organizations can neither solve all their analytics infrastructure problems simultaneously nor culturally shift overnight. So the PoC should be designed to:
- Focus on a specific business problem, driven by lines of business rather than technology groups
- Have clear success measures, agreed upon between all parties as worthwhile and of direct value
- Have the buy-in of specific business groups, with sponsorship from a forward-thinking executive
- Incorporate an ongoing business dialog, so all parties can learn and grow experience together
- Fit with strategic business objectives, so its success will be felt across the broader business
- Be achievable, in that any risks should have been considered and mitigated
You will also have to decide on the deployment model. For example, are you going to work with the big systems integrator (SI) vendors or go on your own with the support of independent solutions vendors (ISVs), cloud partners, or in-house use of open source? Each has advantages, depending on the problem you are trying to solve. Cloud-first models can be difficult to establish in governance-driven industries such as healthcare or nance. In the first instance, we often see customers advancing their analytics maturity based on PoCs that employ spare capacity in their data centers: this additional benefit can be used to promote the initiative with stakeholders.
Step 5: Extend, and focus relentlessly on service delivery and business value
With a successful PoC achieved you will be in a stronger position to create the kind of data platform your organization needs to achieve its long-term goals. You will have developed skills and experience, on both sides of the house, and you will have proven what is possible.
From this point on you will be planning to build on your success. You may want to extend your infrastructure and invest more deeply in other tools in order to bring the benefits of analytics to more of the business. We at Spokes can give you advice about building, scaling, and future proofing an analytics infrastructure, including what solutions to use,
but exactly what you choose to deploy will depend on the data types and workloads you’ve identified. You may need a mix of analytics approaches based on technologies such as the following:
- Solid state storage, enabling significantly faster data processing
- Cloud-based streaming & data management architectures
- Open source tools such as Hadoop* or NoSQL* approaches
- In-memory analytics such as SAS solutions
- Security at the perimeter, access, visibility, and data levels
- Optimized fabrics for data movement between data lakes or warehouses and compute infrastructure
- Advanced analytics tools and frameworks
We know that greater levels of business benefit often evolve quite naturally out of ongoing initiatives. For example, a retailer wanting to better align physical store inventory better with what is in its system could build on this to offer higher-order insights. By tagging inventory with ID codes and using sensors around the store to track them, it could see, for example, where a sweater has been put back in the wrong place, or a spot where a particular dress gets tried on a lot but not purchased. Such real-time insights can be used to identify, then move low-selling inventory.
The IT department can also be data-driven, measured by the amount of business value brought by technology. Being data-driven means closing the loop between cause and effect: learning from what is working in the business and using this to drive greater levels of value and success. As both IT and the business become more experienced in creating data analytics projects, they can start building higher-order analytics capabilities, ordering better services to their customers and partners. For enterprises further along with their analytics initiatives, machine learning and AI represent the next frontier, helping address some of today’s most complex data challenges.
Technology will continue to evolve – right now we are seeing the scale-up potential of in-memory technologies converge with the scale-out of commodity hardware, making it easier to future-proof technical architectures. Even as emerging technology disruptions such as AI and 5G networks make data more untethered, more distributed and ultimately more critical to business success, organizations can get themselves on the journey in the knowledge that their investments will be protected.
We are in the digital age. Organizations that might not think of themselves as such are becoming analytics and data companies. Realizing the benefits of technological change requires that the business and IT teams learn how to work together on specifically focused analytics initiatives. Data-driven organizations see data and analytics as core to every aspect of the business, and we can think of no better platform for success.
How Spokes Digital Can Help?
“Spokes” is among Top-20 Digital Transformation Partners – CIO Review”.
We can help you identify the ideal E-commerce & IT Transformation strategy and partner with you to choose the right solution enabling you to achieve such results as reducing media & IT costs, increasing productivity, and focusing on revenue growth.
Our platform TrinityX™ defines digital & technology roadmap for E-commerce organizations. It provides an in-depth analytical framework for scoring digital maturity, building a phase-wise road map for digital marketing & technology investments required for success.